.avif)
Folding Data #34

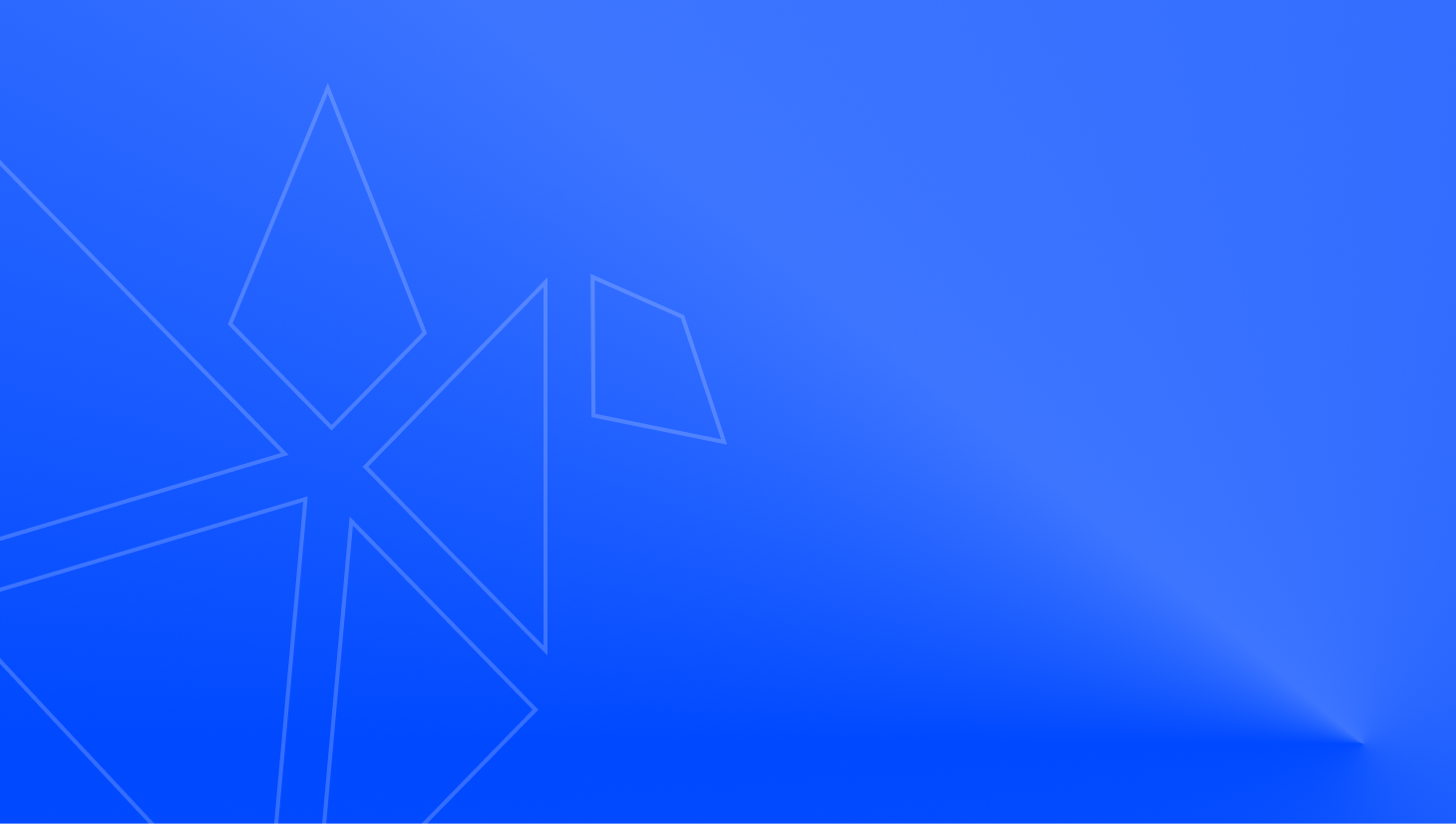
Data Observability vs. Data Quality and why leftists win
Several data quality vendors provide monitors on live data in a warehouse and send an alert if anything looks anomalous. This implicitly means that data must first break before we can fix it. While it does minimize the problem it doesn't solve it. Plus data in warehouses is now being used to power not only internal reporting, but external uses such as email marketing segmentation or data science applications. Data quality issues can have real consequences, and detecting them post-factum may be too late.
In David’s article he suggests that companies should address issues as far left in the data lifecycle as possible. And lays out Datafold’s main use case (without knowing that we solve this exact problem), suggesting companies need:
“Testing dev/test environment data against prod to ensure that merges to a codebase don't damage data quality.”
This is exactly what Data Diff does, glad to see more folks coming around to fixing problems before they are in production.
Data Observability vs Data Quality
Interesting read: Locally Optimal
A/B testing and experimentation has been the holy grail of big tech, with major players boasting their homegrown experimentation platforms running 1000+ concurrent experiments. But maybe the constant search of ever better incremental improvements is only locally optimal in the long run? What if it leads to a general plateau if not stagnation of the business?
“Twitter has used all the standard A/B testing approaches to gradually improve the product over time and the net result is a stagnant number of users and revenue.” – argues Sean Taylor who led data science research solving some of the hardest problems at Lyft (balancing two-sided taxi markets across time and geographies while making some profit is no cakewalk). And Sean’s thought-provoking essay leads us to ponder about the potential and limits of data science in decision making, the nature of disruption and whether generative AI can replace Product Managers.
A/B testing leads nowhere
Tool of the week: Avo
We use Avo at Datafold to define and instrument analytical events. Having spent years maintaining messy even tracking plans in Spreadsheets, I was immediately bought in Avo’s value prop. And indeed, it proved very useful in keeping Analytics and Engineering in full alignment about tracking and helped us save time debugging and fixing instrumentation. Needless to say, having clean and reliable events make it so much easier to manage the data quality downstream, shift-left wins again! Adopting Avo felt as good as moving from Jira to Linear ;)
Events done well go a long way
Before You Go
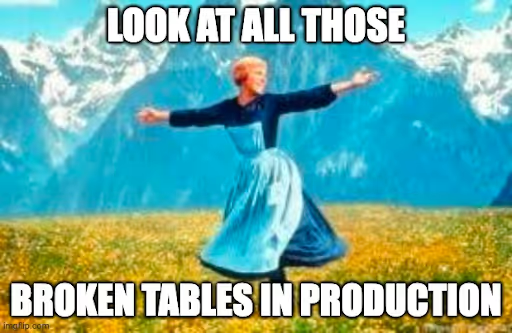
.avif)
