.avif)
Folding Data #10

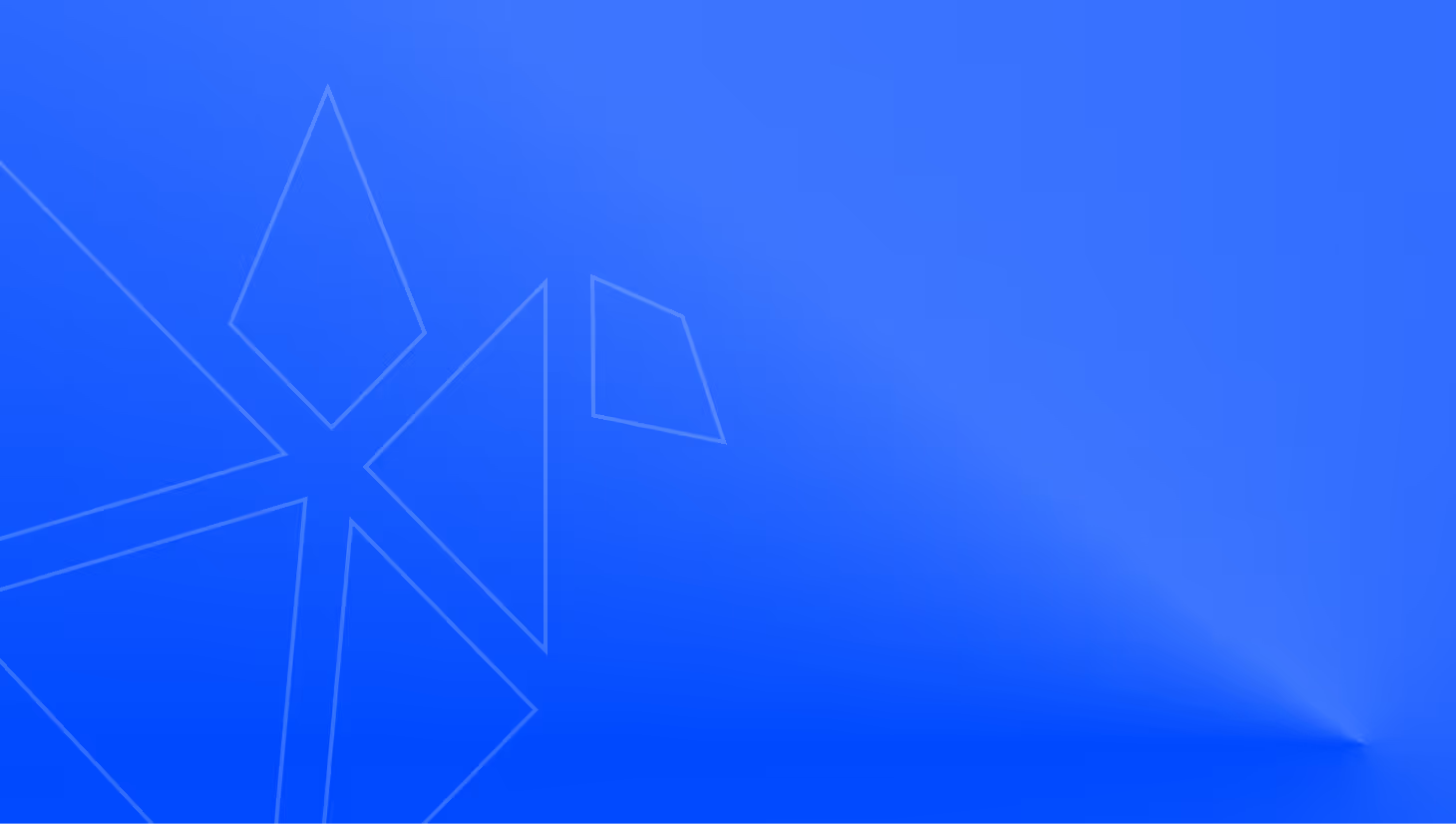
Thank you to everyone who joined our Data Quality Meetup last week. If you forgot to register or attend, not to worry - you can watch the replay on YouTube.
In data quality, there are still far more questions than answers, and the meetup gives us all a chance to share and learn from each other. If youâre interested in giving a lightning talk or sharing your opinion on the panel, please reply to this email and let me know!
Tool of the Week: Evidence
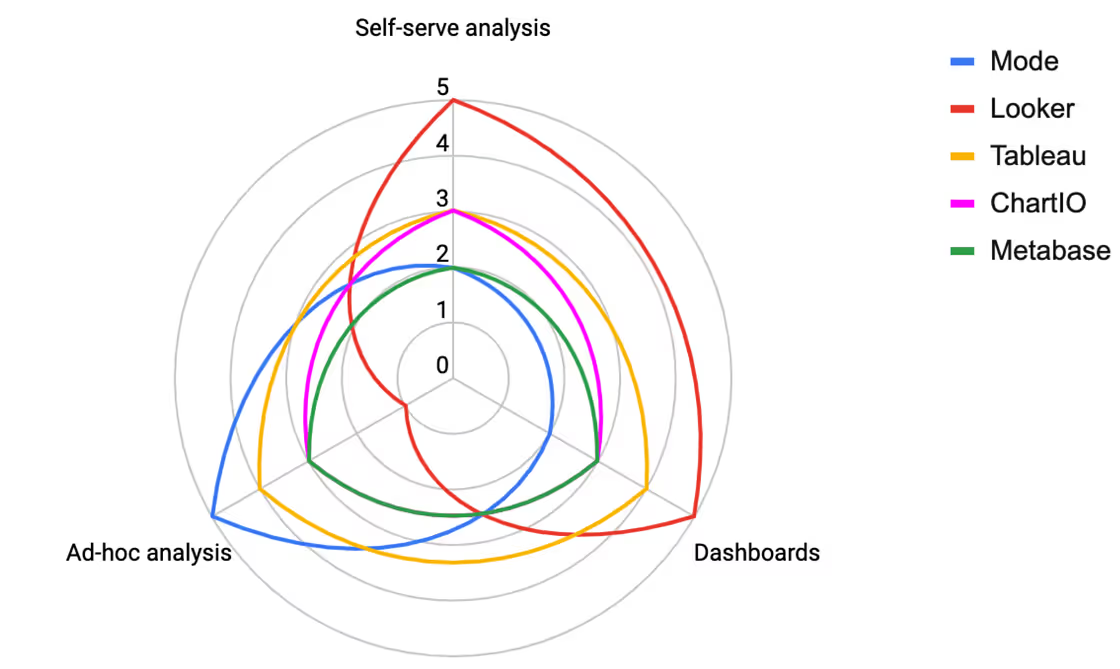
In my earlier post on Modern Data Stack, I categorized BI tools into three main use cases: self-serve, ad-hoc analysis, and dashboarding. Having a tool that excels at each is a dream and will remain such since each use case has its own workflow and optimizes for different things: e.g. structure for self-serve vs. speed for ad-hoc deep dives. For a while, there weren't many good options for ad-hoc, SQL-centric analysis besides Mode and a few Jupyter-influenced tools such as Hex.
Until Evidence, a fellow YC company launched on Hacker News last week. Evidence's approach and differentiation can be described with one word: simplicity. In a world of convoluted interfaces and ever more complex visualization types, Evidence stands out since you only need two things to build a beautiful data narrative with it: SQL and Markdown, languages well familiar to any modern analyst. Needless to say, version control is a breeze with such a paradigm, and if you weren't sold already â Evidence is open source!
An Interesting Read: Modern Data Experience
While we argue over what should or shouldn't be considered part of the modern data stack, get excited about the new ultra-focused niche tools and fight over what product is the best in each category, we often forget about the essential aspect of the data stack â the end-user experience. In his well-articulated post, Ben Stancil reminds us of the common failure patterns, draws historical analogies, and suggests 9 criteria to aspire for both vendors and those who assemble data platforms in organizations.
What I am Proud of
In my days as a data engineer, moving fast (writing a lot of SQL to build and evolve data pipelines) meant breaking things regularly. If you didn't want to break things, you were bound to countless hours of meticulous manual testing.
At Datafold, we've been trying to figure out how to help data teams escape this vicious dichotomy: move fast while being fully confident in the quality of the data they are shipping to stakeholders.
One year ago, we implemented Datafold with our first customer, the awesome Data Team at Thumbtack. A year later, having automatically validated over 1K pull requests for the team's SQL repo, we co-authored a case study showcasing how the Thumbtack's Data Team achieved data quality peace of mind and freed up a host of time that has since been spent on creative and enjoyable tasks as opposed to checking and fighting data breakage.
Before You Go
Over on Reddit, a thread in r/dataengineering should come with a serious cringe warning. The prompt was simple, but the responses might make your heart sink. When you say âTrigger a data engineer with one sentenceâ, these responses are winners (or are they losers)?

.avif)
