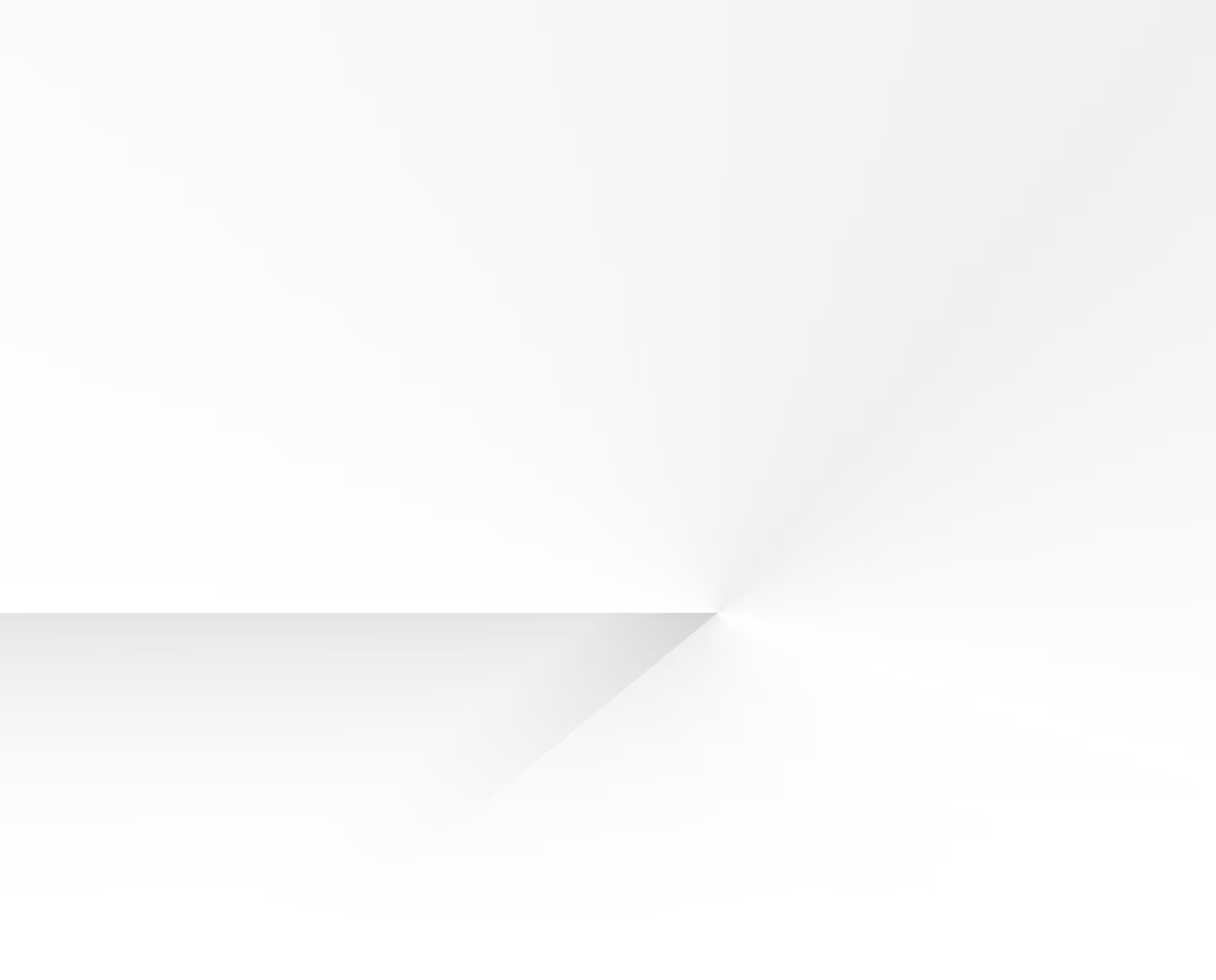
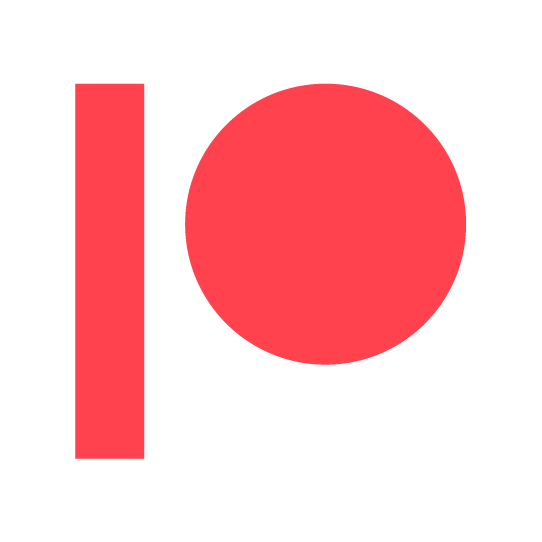
Patreon reduces data incidents and expands data democratization with Datafold
Patreon is a platform that helps creators earn money directly from fans. Over 200k creators across 180 countries are supported by 8 million patrons. Having raised $413.3 million, Patreon is valued at $4 billion and processes over $100m in payments per month.
Introduction
Patreon is a platform that helps creators earn money directly from fans. Over 200k creators across 180 countries are supported by 8 million patrons. Having raised $413.3 million, Patreon is valued at $4 billion and processes over $100m in payments per month.
Datafold's column-level lineage gives confidence in the whole system. If my stakeholders ask “why is this dashboard out of date?” I can answer in 25 seconds instead of digging through pull requests for hours. As a product owner, I can understand how the rest of the company makes decisions based on the data we produce. It brings data confidence and visibility to the company.
The Problem
Patreon had invested in data science early in the company’s history with data robustness and quality as KPIs for the Data Science team. Progressing towards a higher level of data and organizational maturity, the organization continued to be committed to the journey of increasing data quality. Potential public scrutiny of reporting in the future meant that trustworthiness and security were even more vital as the Data Team planned for Patreon’s future.
While the data was in a decent state, new products and business logic introduced some challenges as the company scaled. At inception, Patreon only accepted USD for payments, and then evolved to accept all currencies. This resulted in a big migration for the payment tables, changing and adding columns, plus concerns about making sure that this didn’t impact historical data. This transition meant touching critical data systems that power 80% of the data used across the company.
Finally, in recent years, the team had experienced a few outages in vital dashboards. The issues came from complex changes in the underlying SQL code that was over 400 lines long. While the incidents weren’t frequent, they were enough to cause anxiety for the data leaders, prompting the team to look for a new solution.
The Solution
After evaluating alternative vendors in the data quality space, Patreon decided that Datafold’s solution would be more targeted and strategic, fitting their use case of focusing on proactive data quality assurance rather than running triage on problems in production. In less than a day, the Patreon team was up and running with Data Diff, using the Datafold platform.
Using Data Diff to proactively assess the impact of every change to data pipelines and to identify regressions before they affected production, the Patreon Data Team was able to ensure the high reliability of their data products.
As the Data Science Team received most of its source data from the Software Engineering Team through analytical events or production database replicas, data quality incidents happened when software engineers developing the app made changes to their systems that impacted data science products. While software engineers care about data quality and tended to alert the team if schemas change, the reality was that many changes went unnoticed and data scientists would have to dig into changes and the investigate root cause.
Before Datafold, this caused lots of frustration when pipelines would break and the data team wouldn't know why. They’d have to look through vast amounts of pull requests (PRs), find what removed columns to get the engineer to bring back the column, or write new code to work around it. Sometimes, the Data Team didn't catch these things for days or even weeks.
After a few months of using Datafold, the team adopted additional features from the platform, including Catalog and column-level lineage to improve knowledge transfer and holistic data pipeline understanding. Now all teams can easily see whether and how the changes they are making would affect data science products and prevent data quality incidents from happening, not just reacting to what was already broken.
The Results
- Reduced rate of data incidents. The primary reason for seeking a solution has been addressed, with Patreon less prone to data outages or incidents and improved overall uptime for data products.
- Unified understanding of data. Using Datafold's feature, Patreon was able to consolidate data documentation that had been dispersed across multiple tools and documents and often lacked important context about lineage or data distributions. This dramatically improved the KPI of % of tables documented. The Patreon team is now more aware of the data they have available and how it is in use across the business.
- Improved data democratization. Stakeholders wondering if a column such as “pay incoming” is in USD or a local currency, or if the amount includes taxes, can easily look at the column in lineage to answer the question themselves without needing to ping a data scientist to ask where the number is coming from. This both improves stakeholder buy-in regarding data and increases morale and productivity for data scientists.
- Streamlined data hire onboarding. The Patreon team uses Catalog and during onboarding so that new analysts or engineers can easily see how tables are built and where the data originates. This results in faster data science onboarding and knowledge transfer.
Column-level lineage gives a holistic view of data dependencies and interdependencies. It’s so powerful - with even more insight than table-level lineage - I get really excited about what it can do!